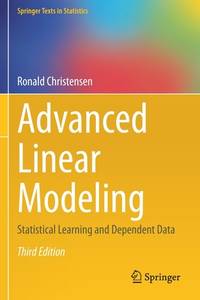
Stock Photo: Cover May Be Different
Advanced Linear Modeling: Statistical Learning and Dependent Data (Springer Texts in Statistics) Paperback - 2021
by Christensen, Ronald
- Used
- Good
- Paperback
Drop Ship Order
Description
$123.98
FREE Shipping to USA
Standard delivery: 7 to 14 days
More Shipping Options
Ships from Bonita (California, United States)
Details
- Title Advanced Linear Modeling: Statistical Learning and Dependent Data (Springer Texts in Statistics)
- Author Christensen, Ronald
- Binding Paperback
- Condition Used - Good
- Pages 608
- Volumes 1
- Language ENG
- Publisher Springer
- Date 2021-01-08
- Illustrated Yes
- Features Illustrated
- Bookseller's Inventory # 3030291669.G
- ISBN 9783030291662 / 3030291669
- Weight 1.93 lbs (0.88 kg)
- Dimensions 9.21 x 6.14 x 1.28 in (23.39 x 15.60 x 3.25 cm)
- Dewey Decimal Code 518
About Bonita California, United States
Biblio member since 2020
From the publisher
From the rear cover
Now in its third edition, this companion volume to Ronald Christensen's Plane Answers to Complex Questions uses three fundamental concepts from standard linear model theory--best linear prediction, projections, and Mahalanobis distance-- to extend standard linear modeling into the realms of Statistical Learning and Dependent Data.
This new edition features a wealth of new and revised content. In Statistical Learning it delves into nonparametric regression, penalized estimation (regularization), reproducing kernel Hilbert spaces, the kernel trick, and support vector machines. For Dependent Data it uses linear model theory to examine general linear models, linear mixed models, time series, spatial data, (generalized) multivariate linear models, discrimination, and dimension reduction. While numerous references to Plane Answers are made throughout the volume, Advanced Linear Modeling can be used on its own given a solid background in linear models. Accompanying R code for the analyses is available online.
This new edition features a wealth of new and revised content. In Statistical Learning it delves into nonparametric regression, penalized estimation (regularization), reproducing kernel Hilbert spaces, the kernel trick, and support vector machines. For Dependent Data it uses linear model theory to examine general linear models, linear mixed models, time series, spatial data, (generalized) multivariate linear models, discrimination, and dimension reduction. While numerous references to Plane Answers are made throughout the volume, Advanced Linear Modeling can be used on its own given a solid background in linear models. Accompanying R code for the analyses is available online.